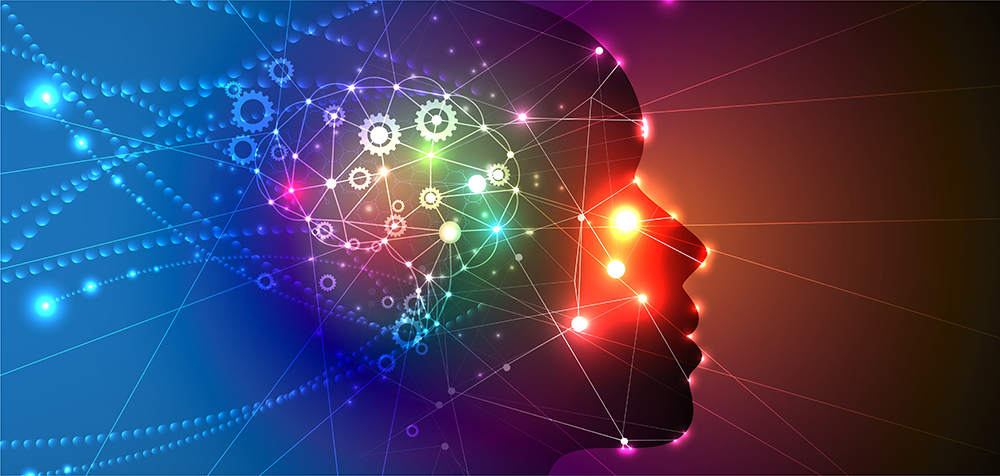
In the ITS world, we hear a lot about artificial intelligence (AI) and machine learning (ML) in general – not least for their role in functions such as traffic management and various aspects of smart cities. In the Weigh in Motion (WiM) sector specifically, there is perhaps slightly less chatter. But AI is already being used in WiM – just not always in the way you might assume.
“The future looks bright for the incorporation of AI in WiM,” suggests Rish Malhotra, CEO of International Road Dynamics (IRD). “There will be opportunities to develop and deploy new types of systems with faster roadside computers for edge and/or cloud applications. It is quite likely that most sites will use AI in some fashion as they upgrade and as fast network communications proliferate.”
For Christoph Klauser, business development traffic solutions at Kistler, AI “is a great technology with lots of potential for WiM”. He thinks there will be two main benefits: AI can be used to increase WiM accuracy – and also to increase confidence in WiM results, through the better detection of irregularities and discarding of invalid measurements.
In fact, Vaclav Blahnik, product manager of telematics at Cross Zlín, points out that AI is already an “integral part of our WiM systems”. “Not that it is directly involved in the weighing, but it is part of a subsystem that performs LPR recognition based on video from the cameras,” he explains.
Malhotra cites promising uses for AI that IRD is pursuing, “including improving WiM accuracy with continuous calibration and compensating for external factors including vehicle dynamics that typically affect WiM measurements”.
The Canadian firm is investing in and developing new AI applications in commercial vehicle enforcement (CVE) systems integrated with WiM - and has been working on various inspection technologies, most notably a CVSA decal reader for automatic visual verification of vehicles’ inspection status.
Fewer sensors
“Driver compliance systems that identify driver behaviour issues such as use of cellphones or seatbelts may be integrated with WiM pre-screening in automated weigh station systems,” Malhotra goes on. “Using AI for re-identification of commercial vehicles allows CVE systems to operate with fewer intrusive sensors, enabling an increased bypass of pre-vetted vehicles travelling on designated transportation corridors.”
A couple of years ago, IRD launched its iTHEIA vehicle count/classification product, capable of accurately classifying vehicles into a standard FHWA 13-class scheme at high speeds in a multi-lane environment, Malhotra adds. “It demonstrates that AI-enabled, video-based classification will play a role in augmenting or replacing in-road sensors for data collection and toll applications.”
While VanJee does not yet apply AI in the core functions of its WiM system – such as measurement and weighing - it does use AI elsewhere, says chief scientist Wang Ping. This includes in WiM malfunction diagnosis (including sensors which are broken or not operating normally, or whose output changes), collecting vehicle axle numbers, assessing whether tyres are single or dual, vehicle classification and the identification of irregular driver behaviour such as acceleration, deceleration, oblique movements and pauses.
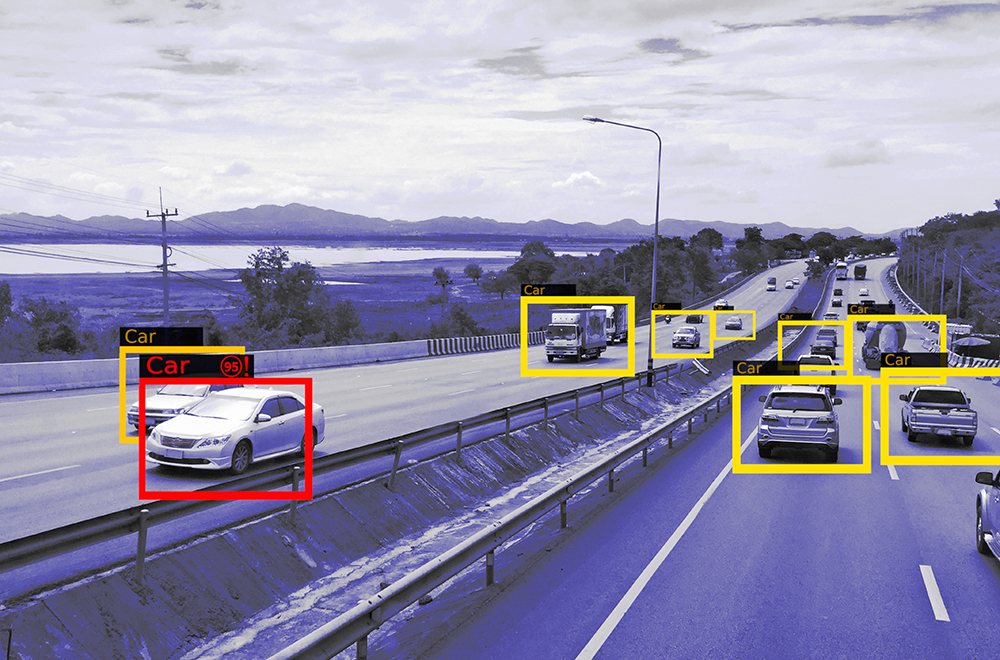
“We do know that with WiM, the output of the in-road sensors, whether they be piezo electric or piezo quartz, are directly affected by the temperature of the road body to varying degrees,” says Andrew Lees, Q-Free WiM business development manager. “As a result, a number of systems have automatic compensation algorithms built in which monitor the road body temperature and automatically compensate for the outputs produced by the in-road sensors to maintain a consistent level of weight throughout the temperature ranges. This is achieved by ML algorithms.”
However, he adds: “The biggest issue in WiM at the moment is addressing direct enforcement applications. If this is the main driver behind the industry then I feel anything that is based on vehicular telematics or machine learning that cannot be openly declared and proven in a court of law is unlikely to be introduced.”
Florian Weiss, CEO of Traffic Data Systems, believes there will be a further place for AI in future. But he also believes that there is some way yet to go: “I think all manufacturers of WiM are still facing problems in doing proper measurements on what I would say is a ‘lower level’.” Those problems need to be dealt with “before we can really say we can identify outliers so well that we can go for the next step in order to reduce the measuring error any further by using AI”.
Wang Ping highlights three major ‘bottlenecks’ for AI application in WiM. “Few WiM companies have established adequate WIM datasets for AI,” he says, since this involves hundreds of sites, with thousands of sensor signals at each one, with corresponding real vehicle weights. The second issue is that due to AI algorithms’ high level of complexity, “there are few WiM companies putting resources into research and development of AI in WiM applications”. And finally, “there is a contradiction with AI algorithms and metrological code”.
AI expansion
Despite these issues, Vaclav Blahnik at Cross Zlín insists the use of AI is ‘definitely’ expanding in WiM products and solutions: “It is mainly in the detection of vehicle parameters - detection of the raised axle of a truck when validating a vehicle overload violation; detection of the type of load, where, for example, a tanker cannot be reliably fined, because the fluid transported during the journey constantly changes the distribution of the load on the individual wheels and axles.”
AI technologies are being considered to augment work done by human inspectors to ensure safety concerns are not overlooked “in a CVE landscape now typified by increasing traffic and enforcement personnel shortages”, Rish Malhotra suggests. “There are also opportunities to deliver less intrusive and more accurate traffic data systems. As vendors and agencies become more comfortable relying on AI/ML-powered systems, we expect their use will expand.”
In fact, despite the caveats, there is general agreement on the future potential for AI in WiM. “There have been a number of academic papers addressing this issue over the years and there has been one study suggesting the use of vehicular telematics,” says Lees. “This is used to analyse driving behaviour and road anomalies and would be combined with ML techniques. Obviously, this is not a simple process as the learning process is carried out by analysis of the data rather than specific programming. I have to use the phrase ‘predict’, as machine learning uses a variety of algorithms that iteratively learn from the data to improve, describe data and ultimately predict outcomes. If this is the case then it can only be used for maintenance, protection of the infrastructure and statistical purposes.”
Lees cautions that companies also have to consider the base data that is being used in the machine-learning process. “It has to be accurate otherwise you are going to end up with the classic data analysis scenario of ‘garbage in, garbage out’,” he says. “To ensure your base data is accurate, the system providing this data needs to be monitored meticulously and quite frankly this could become a huge drain on resources of time and money. I’m not saying I’m against it, I’m just trying to be practical.”
Kistler’s Christoph Klauser agrees that there is much work to be done. “Currently big datasets with annotated, verified data of excellent quality is needed to train AI algorithms,” he says. “The big challenge for WiM systems is to know the ground truth about the static weight and the actual driving behaviour of passing vehicles. The better we manage to get such annotated datasets (matched data with verified static weight and dynamic weight and indication on driving behaviour), the better we can benefit from AI.”
Malhotra remains upbeat. “In the near term, AI will enable more efficient commercial vehicle enforcement by automating inspections, improving safety, and improving the accuracy of WiM systems,” he insists. “In the longer term, there is potential for direct enforcement applications including automated issuance of citations.”
Predictive models
AI and machine learning will also have applications when upgrading current WiM systems by predicting sensor degradation and calibration drifting with the goal of providing higher confidence in weighing accuracy, he continues. “From a regulatory perspective, this will ensure results are trusted and auditable. Predictive models will help in determining allocation of resources for processing overweight vehicles. Historical data mining will identify trends that will allow automated stations to implement smart queuing at the ramp, lowering risk of accidents. Automated inspection will advance and improve automated screening for maintenance and safety issues that may be identified by AI and machine vision systems or by trusted, connected vehicle systems.”
At Cross Zlín, Vaclav Blahnik says: “Previously, it was enough when the vehicle was weighed and there was information about whether the vehicle is overloaded as a whole. Today, it is necessary to know the load distribution of individual axles and wheels. In practice, this means more accurate detection of forces from individual wheels using sensors, but also more accurate classification of vehicles in terms of vehicle type, load, vehicle use, etc. The diversity of classification is theoretically infinite and commonly assessed in criminal proceedings. At today’s traffic density, the number of vehicles passing on motorways, this is no longer in human power. So devices must also enter this ‘industrial’ sector. These devices are software devices, i.e. AI, that are able to replace humans.”
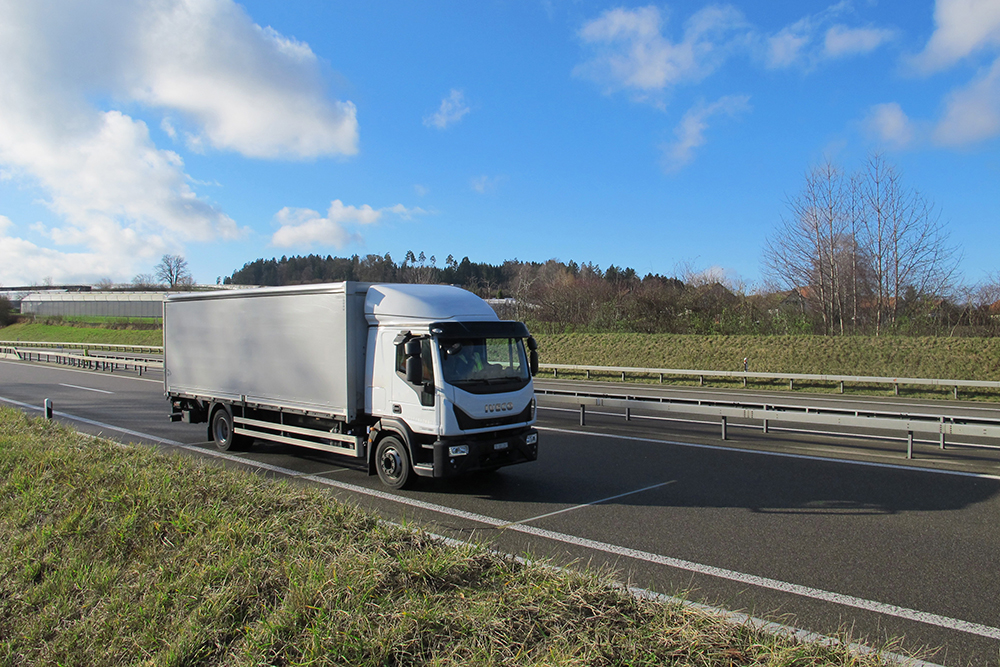
Lees takes the ‘never say never’ line but reiterates: “Legal enforcement is probably the main driving force behind the industry at present, so I cannot see room for AI as it stands when addressing legal applications. As we all know WiM has so many variables that need to be taken into account when carrying out measurements that predicting weights is unbelievably complex.”
Things will inevitably improve, says Klauser. “The more data a WiM system can provide during the crossing of a vehicle, the better AI can help to find patterns which really bring benefits to the end customers.” He cities Kistler’s new digital platform, KiTraffic Digital, as a starting point “for this long journey to benefit from AI in the upcoming years”.
Much more data is available compared to existing analogue WiM sensors, he explains, and AI algorithms can be applied on multiple levels: “On a WiM sensor level or WiM system level or even on a solution level, where camera or dimension scanner data can be included.”
For Wang Ping this all adds up to the potential for a “revolution“ for the WiM industry via AI. And Rish Malhotra at IRD agrees: “AI will greatly benefit the world of WiM through improving accuracy, efficiency and ease of use for transportation professionals and commercial vehicle enforcement agencies. Before long, AI will be a routine part of WiM system implementations.“ ITS