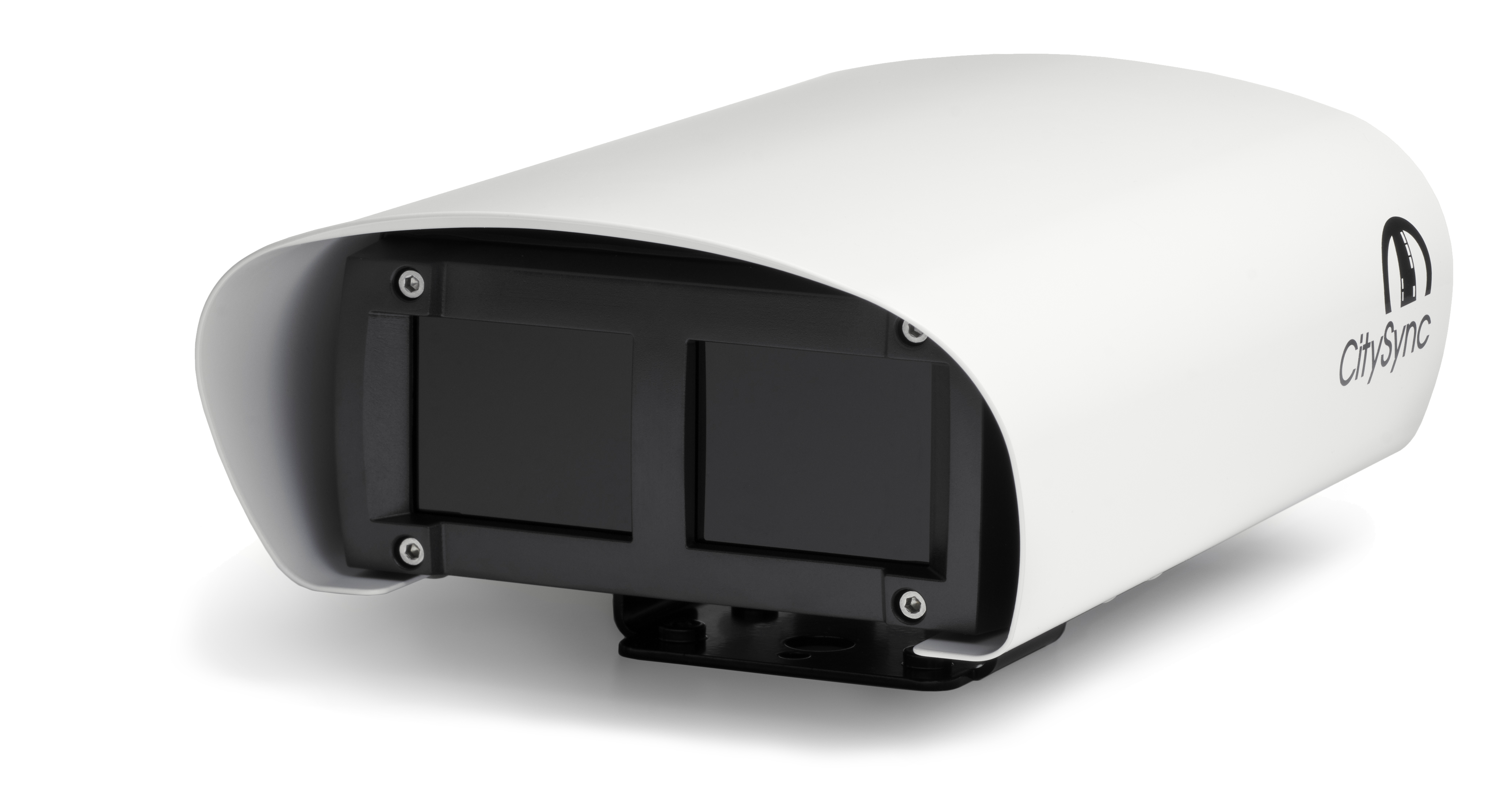
The sheer range of variables makes it difficult to find a single algorithm to ensure a 100% standard of ANPR. David Crawford investigates new processing technology.
Automatic number plate recognition (ANPR), using optical character recognition and image-processing to identify vehicles, plays key roles in traffic monitoring and law enforcement, access and parking control, electronic toll collection, vehicle security and crime deterrence. Overall, system performance is well rated, with high levels of accurate captures.
But gaining consistently optimal results can be subject to factors including high vehicle speeds, lighting and weather conditions, use of non-standard plates and the spacing of characters. At the same time, growing capabilities to handle high-definition images are delivering greater accuracy – a major advantage when segmenting plate content – and contributing to the continuing development of artificial intelligence-based and ‘all-in-one’ systems.
Crucial pixels
Accurate plate detection within a larger image of a vehicle is crucial. As a recent study by India’s Savitribai Phule Pune University stresses, this substantially lowers the cost of, and time needed for, subsequent computation and also reduces the complexity of designing the algorithms necessary for extracting the relevant data. A typical 1024x768-resolution image contains 786,432 pixels, of which the number plate itself will typically account for only 10%.
One method widely used in plate location optimisation involves edge detection, which scans and highlights the boundaries between an object within an image and the overall background. It uses mathematical tools to identify points where there are discontinuities - or abrupt variations in pixel intensity - joins these points and then compares the result with a recognisable conventional shape – for example, that of a vehicle number plate.
In the process, it filters out and discards irrelevant information, while preserving key structural properties and enhancing specific features.
A recent project carried out at the University of Hertfordshire in the UK involved developing edge detection-specific algorithms and combining these with connected component analysis. The technique takes advantage of the fact that each character on a plate consists of a group of pixels, all in contact with each other.
This approach has substantially improved real-time plate detection rates, using a digital signal processor for carrying out mathematical manipulation. The complex process of defining what are ‘good edges’ involves eight distinct parameters to reduce the risk of erroneous identification and wasted processing time.
Algorithm difficulty
The researchers used, as their raw material, 45,000 plate images, a higher number than that achieved in most previous exercises, to provide a wide enough sample to reflect prevailing conditions on busy roads at speeds of up to 125km/h. The resulting range demonstrated the difficulty of finding a single algorithm capable of ensuring a 100% standard. An early conclusion was the importance, for obtaining accurate reads, of the character spacing on licence plates, indicating the need for more work to highlight the precise impact of unsatisfactory juxtapositions in generating partial misreads or complete failures. Some characters are commonly confused because of their physical appearance (see table 2).
Globally, the situation is complicated by the fact that each country (and often region of a country) has its own, sometimes loosely defined, standards for number plate sizes, positions, fonts and spacing. These enable a range of formats with wide variations in the way that alphanumeric symbols are placed on sometimes complex backgrounds that allow for local expressions of identity. Stacked letters (with one placed above another) add to the read issues. In the US, the range of possibilities exceeds 6,000.
Meanwhile, ANPR system vendors naturally have clearly defined conditions for guaranteeing high levels of accuracy in detection and recognition. A plate at or close to the limits of these may not be detected or recognised, even though it is perfectly readable by the human eye.
Recently available solutions are streamlining the whole process by deploying technologies including edge detection ahead of post-processing. Location of the technology within the ANPR unit itself can work satisfactorily on vehicles that are stationary (for example parked), or moving slower than 24km/h.
Moving faster
Where vehicles are moving faster, an intermediate server, ideally with a graphics processing unit, takes the strain. Combining deep learning and machine learning techniques, the system has achieved 99% success rates in the US and other countries. The software is ‘trained’ on thousands of images of plates to recognise content in poor lighting or bad weather and in irregular formats. Another issue that has previously been little researched concerns plate materials. A two-month UK study of vehicles with standard-size fixing screws (or caps) fitted within the letter ‘U’ generated nearly 1000 captures with a misread rate of 28.5% - well above that of all the other factors (see table 01). Replacement with smaller diameter anti-tamper screws reduced the footprint of the fixing by 75% and brought the failure rate down to 0.3% - in addition to giving greater security. Lacy-Thompson insists: “Our system is taught to ignore spurious marks and fixing screws.”Again, there is the risk of absorption of rear fixing adhesives by the number plate’s retroreflective layer, with consequent impacts on the quality of images. Chris Glendinning, group export director of Hills Numberplates, says: “ANPR is becoming more heavily relied on across a range of markets. But even a system offering industry-leading accuracy will be heavily compromised if plates are physically difficult to read - or if there is a high risk of counterfeiting or cloning. This highlights the importance of maintaining security and quality control throughout the whole supply chain, from raw materials, through people that access and use them in manufacture, to delivery of the completed plate to the end user. All these stages can be effectively controlled if the responsible national authority is focusing on the plate in the context of its operation with ANPR technology”.
The University of Hertfordshire has identified manufacture as a key area for future study, along with the implications of ensuing action by countries’ national legislatures.
Parking problems
In a typical parking scenario, conventional ANPR can normally detect and correctly identify around 97% of vehicles. But the ability to reach the highest possible level is important for ensuring maximum revenues (and minimising the risk of issuing incorrect tickets).
One way of enhancing success rates is to combine standard ANPR data with confirmatory output from additional vehicle presence detectors that can also be installed inside the camera. These can use, for example, edge detection technology to capture a recognisable image (and the location) of a suspect vehicle even if its number plate is missing. The system transmits the evidence of any irregularity in a management report for human follow-up.
In June 2017, Swedish firm
in revenue is then in the region of €13,500 (US$15,750) per day.
Taking the additional security benefits into consideration, this gives a compelling reason for including vehicle presence detection.”