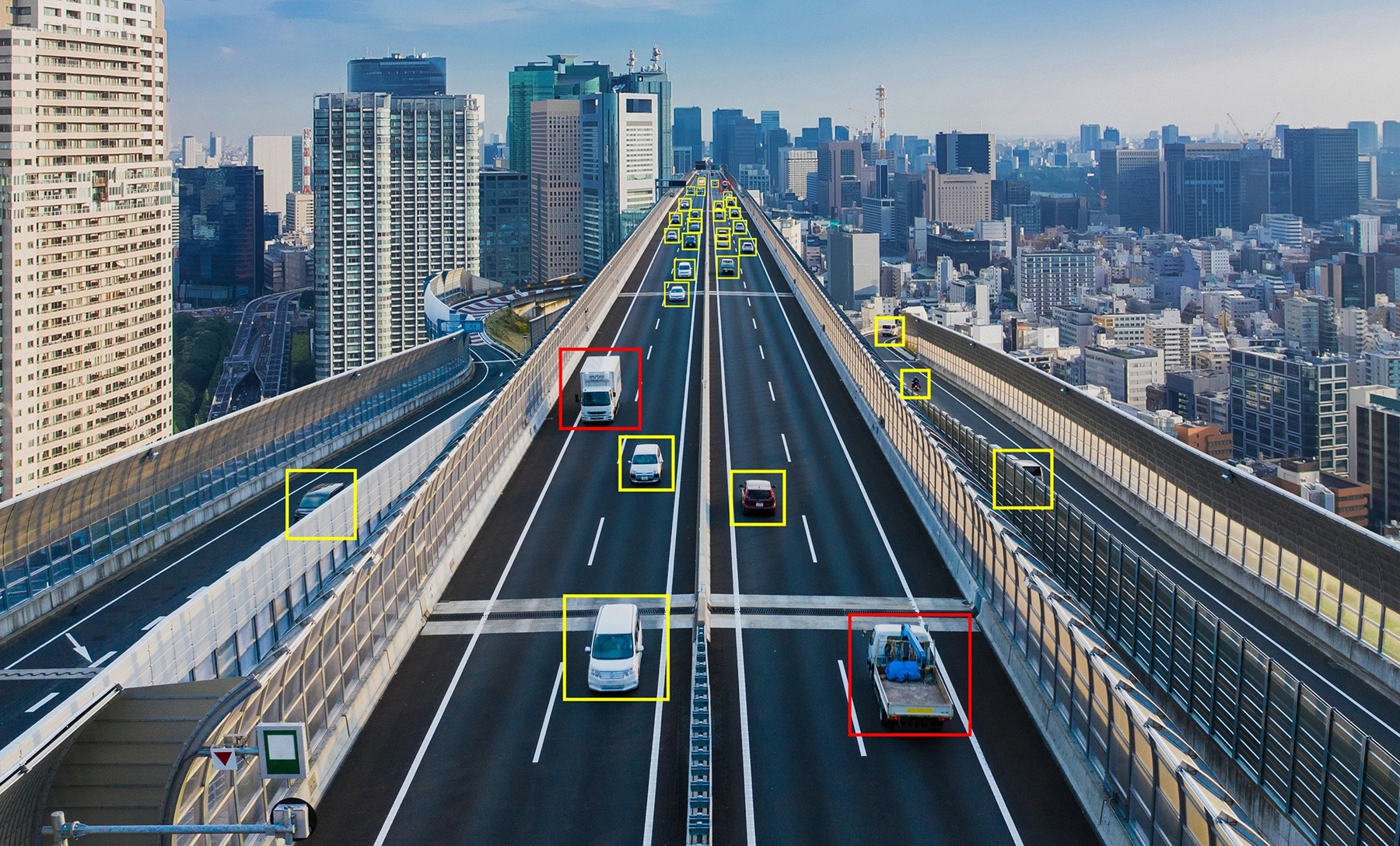
The world is seeing an acceleration in the deployment of smart cities. However, these have been slower than expected or even scaled back, often facing backlash. Even the definition of what constitutes a smart city has been changed, relative to concepts from 15 years ago when flying vehicles were foreseen. This article will discuss smart cities from the viewpoint of traffic management, which has proven to be one of the most challenging implementation areas.
Benefits and challenges
Promises of increased safety, less pollution, increased productivity and a better quality of life in smart cities are just too good to be ignored. A city is a much more complex system than most people realise. Earlier expectations likely needed to be tempered. A good example is the congestion pricing programme in New York City, in which most passenger cars were to be charged $15 a day to enter a congestion zone below 60th Street in Manhattan. This contentious plan has been years in the making and was set to come online this year before New York governor Kathy Hochul’s decision to pause it indefinitely.
Either way, it exemplifies some of the challenges faced with traffic management systems. Let’s review some of the main challenges of traffic management in smart cities:
• Large scale The concepts of smart cities are best applied to larger urban areas. It is difficult to segment the deployment of a smart city project into a smaller, phased approach. Smaller deployments are fine for proof of concept, but not so much to realise the full benefits of what a smart city could be.
• Interconnected The real magic comes from diversified sensors, interconnected and working as an intelligent single entity. The connectivity between these sensors can be difficult to achieve from a network point of view, but also for software when each sensor has a different API and different ways to communicate. Smaller, narrowly-focused systems are fine within the limits of what they do but they cannot scale to a smart city system without interconnectivity.
• Everchanging A city is a dynamic, changing space in constant, rapid renewal. Traffic flow gets modified continually because of new developments, road repair, accidents and other occurrences. The drive for densification brings new challenges in updating infrastructure in ways that could not have been conceived of during past urban planning.
• Privacy The concept of smart cities includes privacy risks as even more personal data is now available to commercial and government agencies. The risks of a data breach or system hacking can bring huge complications if sensitive data is exposed.
• Legal and political framework Technology is meant to support society and not the other way around. The deployment of technology with such societal consequences as a smart city must be thoroughly thought out. Legal and political frameworks advance at their own pace, lagging the lightning speed associated with technology development. There are also concerns about smart city data being used for litigation purposes.
Edge architecture
The core promise of advanced traffic management is better traffic flow and reduced congestion. This has proved challenging to achieve. Early proofs of concept have often worked well enough to entice city managers to further explore large-scale deployments, only to be deflated by the complexities of these large-scale systems. One way to reduce the complexity is to process information right at the point of collection using an edge processing architecture.
“Architects of smart city infrastructure must take into consideration the evolving nature of AI”
Edge processing delivers the ability to analyse data at the sensor level. Sensors can be of various kinds including vision-based detection, counting, classification, monitoring and more. Other sensors could measure air quality, weather conditions, road surface and noise level. Edge processing on any of these sensors can reduce data by filtering or even by making decisions locally.
By only transmitting results instead of the raw data edge processing architecture presents many benefits:
• Resilience An edge-based system is more resilient by nature as there is no single point of failure. Systems for smart cities are mission-critical and there are several things that can go wrong.
• Speed Such a system performs faster since it does not have to wait for data to be transmitted and processed remotely before making a decision, possibly facing a bottleneck in the processing queue.
• Scalability An edge-based system can be easily scaled up in size as the need expands. Capacity and coverage can be increased by simply adding more nodes without having to revamp the entire system.
• Privacy Edge processing offers the added benefit of reducing concerns around privacy. This approach minimises the quantity of raw data being exchanged within the system or finding its way to hosted cloud servers.
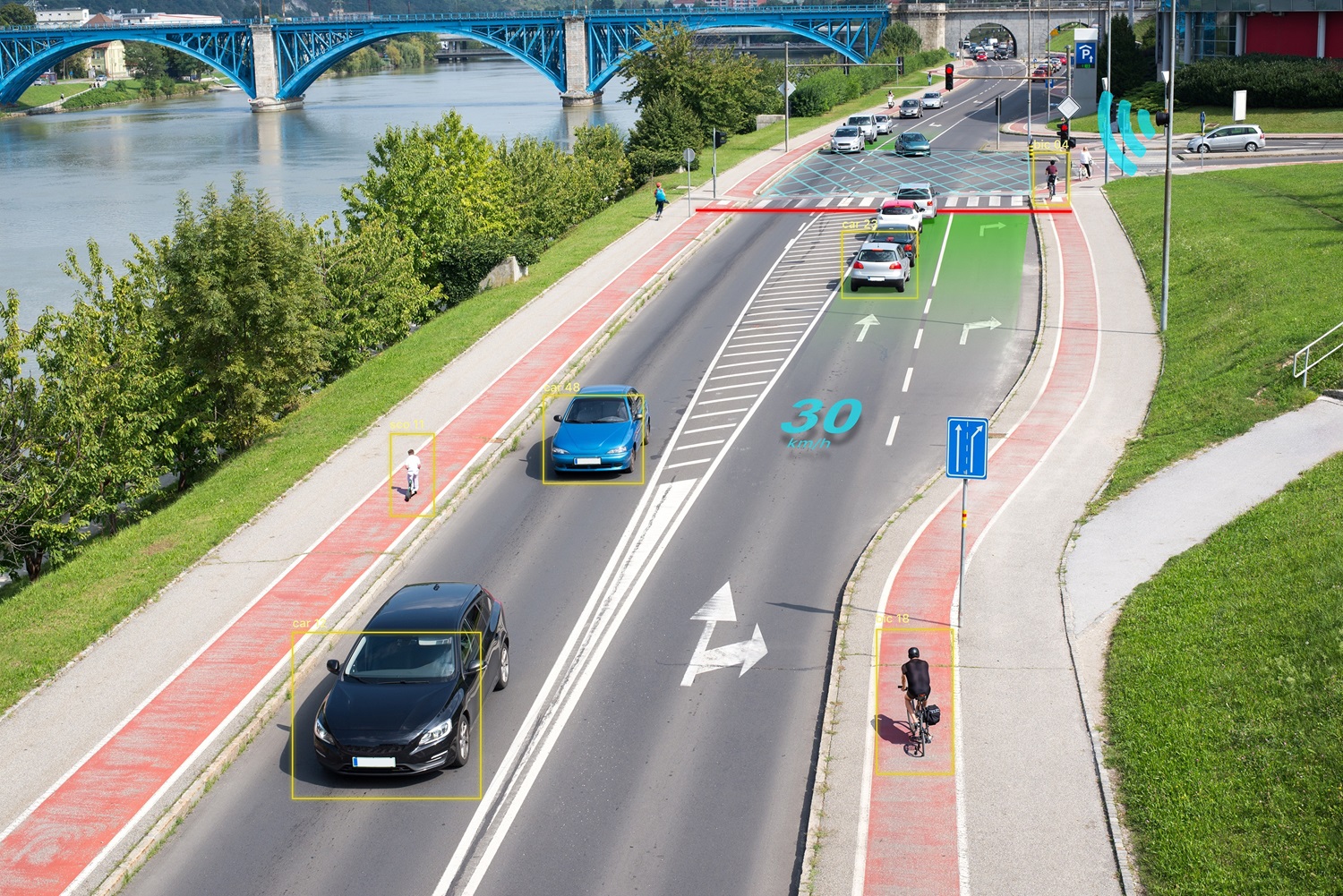
New solutions are emerging driven by combining innovations in edge processing and artificial intelligence. AI enables the merging of information from multiple sensors, identifying patterns and correlations in a way that classical algorithmic programing cannot do. AI is not a new topic and we have been talking about applying it to traffic for nearly 10 years. AI in its earlier iterations might have raised expectations beyond what was possible at the time. However, one of the most exciting features is how quickly it is evolving. Capabilities that were not possible yesterday may seem trivial today.
Architects of smart city infrastructure must take into consideration the evolving nature of AI. The infrastructure needs to be designed with components that are easily upgraded to tomorrow’s version of AI, preferably with software. To accomplish such a task, hardware components - including sensors - must offer flexibility and embedded processing capabilities, potentially GPU- or FPGA-based. In a camera system, that would imply the use of memory and a processing engine of some sort.
Cameras are a critical sensor in a smart city implementation. Cameras could be deployed at intersections, used for monitoring roadway traffic, and function as enforcement devices. To build flexible systems, cameras must also be specified with imaging capabilities that go beyond the immediate task at hand. Characteristics such as high resolution, better dynamic range and faster frame rate can be analysed with a future-looking mindset, allowing a solution to grow over time. Imaging sensors have evolved rapidly in recent years - just like the evolution we see with AI.
Having access to a wide camera portfolio is a way to future-proof a system. Recent advancements in camera technology have led to new features particularly useful for smart cities. For example, the ability to provide synchronicity in a multi-camera system – such as those found at intersections – or the ability to pare down the quantity of information being processed by using in-camera multi-regions of interest.
The way forward
An architecture needs to combine intelligent edge sensors with central host capabilities to fully benefit from new AI capabilities and deliver on the full range of capabilities expected in a modern smart city system. The central host should ideally be deployed through cloud computing which – just like edge computing – is flexible and simpler to scale up. The components needed to build such a system are available today, including cameras with built-in processing or simpler machine vision cameras that are easy to connect to co-located embedded processor boards through USB ports.
“A city is a much more complex system than most people realise”
This implementation strategy brings a new level of capabilities unseen until now, where a system can predict events in real time instead of simply reacting to a condition. Indeed, when a traffic obstruction occurs, the system can do much more than issue warnings and block local intersections. Instead, it can re-adjust traffic flow across an entire neighbourhood by predicting how a local obstruction will impact a larger region. The need for a real-time response and prediction, rather than reaction, is a requirement for modern infrastructure management.
Some of the earlier examples of smart systems deployed in traffic applications were automated speed and red-light enforcement. In a smart city, we can now include much more advanced enforcement systems including distracted driving (mobile phone), dangerous driving (erratic manoeuvres and impaired drivers) and traffic flow obstructions. The high level of connectivity allows systems to communicate in real time with police enforcement and other agencies to provide real-time responses.
Other new capabilities enabled by this new technology include smart parking, dynamic speed limits, multimodal traffic flow management, smart traffic lights and more - all part of a fully interconnected intelligent system. Such a system delivers on the promises of increased efficiency and safety for all road users – from vehicles to cyclists to pedestrians. Ultimately these benefits translate into greener cities and a better quality of life.
ABOUT THE AUTHOR
Dany Longval is vice president of commercialisation at Teledyne Flir