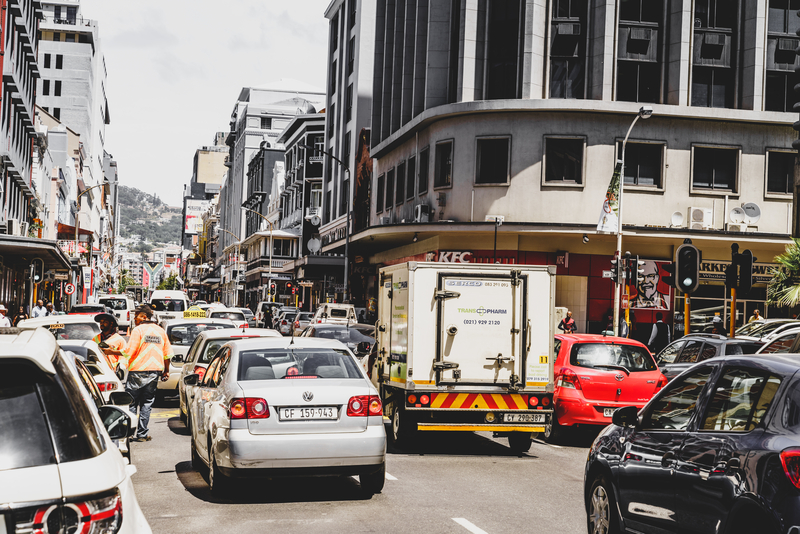
Cepton and Fibre Based Integrations are developing Lidar-based vehicle detection solutions to monitor lane usage and identify vehicles using incorrect lanes in Cape Town, South Africa.
As part of the project, Fibre has deployed vehicle classification systems on traffic lights at intersections and overhead devices that utilise Cepton’s Helius Smart Lidar System with Vista-P Lidar sensors.
Cepton says many of Cape Town's roads have designated bus lanes, and Fibre's Lidar-enabled systems will allow the city technology department to gather real-time lane usage data to enable analytics that help identify ways to improve traffic flow and safety.
The systems will utilise Lidar's perception capabilities to provide information such as vehicle size, traffic volume, frequency, lane positioning and potential obstructions. System operators can then gain an insight about how a specific intersection or piece of road is being used – including unauthorised vehicle types in designated bus lanes, peak traffic time windows, real-time lane occupancy status, wait time at traffic lights and accident-prone areas.
Josh Goosen, system engineer at Fibre, says: “The reason we chose to deploy Lidars in our vehicle detection and classification systems is their superior accuracy. We have previously tested radars and some other types of sensors, and we found that they lack the resolution needed to accurately locate and count vehicles and provide accurate 3D information about their sizes and speeds.
“We found Cepton Lidars to be the best performing and most accurate compared with others we tested, and this has unlocked new possibilities for implementing our smart city solutions with the City of Cape Town.”
Additionally, Fibre and Cape Town are exploring using Cepton's Lidars to augment the city’s analogue and video systems with an additional layer of functionality and performance. The aim is to blend human and vehicle classification, enabled by Lidar, with an artificial intelligence platform to understand how both interact with the city environment.
According to Cepton, this will help collect useful information for traffic management and urban planning through machine learning, while minimising disruption to people’s daily lives.