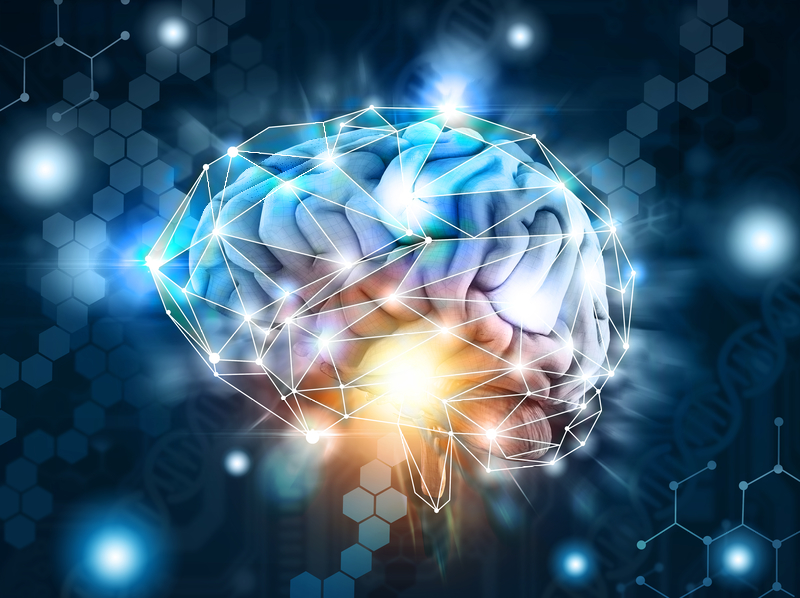
The Information Age has given rise to many society-shaping tools and technologies, nearly all of them revolving around the gathering, dissemination, and analysis of information. Data collection and data analysis technologies have held a careful balance, but as data collection hardware improves and passive data collection becomes more prevalent, it is not an uncommon problem for transportation agencies to have access to more information than is useful in the scope of human analysis through regular spreadsheet programs. Enter artificial intelligence (AI): the nebulous superhero of data crunching, here to rescue a transportation network inundated with data and make some sense of it all.
With all this data lying around, it’s important to make the most of it. Agencies have a responsibility to the public to make their networks safe and efficient. This is difficult to do with tight funding and ageing infrastructure, but data is cheap and plentiful - the problem is that the data is spread out across and within agencies, often held in silos, and few people are in a position to take a wide view of the network. The advent of sweeping AI capable of making long-range decisions is inevitable and necessary for the future of the world’s infrastructure. Implementing AI into the planning process is the only way to create an optimal transportation network in the future, and public agencies should be preparing themselves for that eventuality.
Regional and national AIs are inevitable for several reasons. Thanks to fibre networks and 5G, the information of today’s world moves at nearly the speed of light. It is unreasonable to expect a human to still make the best possible decisions while overwhelmed with all that information. AI has the power to process the flood of data into human-sized chunks or even make optimal decisions by itself, faster and more consistently than any person.
More importantly than the speed, the true wonder of AI is that it can make better decisions and think on a higher plane than human brains. The DeepMind projects AlphaGo and AlphaGo Zero (see box) are clear examples that - when given the correct scope and enough data - the machines can create strategies that even humans who are masters of their craft cannot understand without retroactive analysis. This same high-level perspective and creativity can be applied to solving transportation problems, and find solutions that the engineers and planners wouldn’t have considered.
Data availability
The primary limitation to all AI is the availability of data, which is what makes transportation planning a perfect area for strategic AI implementation. The transportation industry is data-rich and largely controlled by government organisations everywhere in the world. This makes data collection easier to standardise and provides more rigid boundaries and defined scopes than many other use cases. A unified, holistic view allows planners to design for sustainability, address environmental and social problems, and maximise the available resources even in a flagging economy. As long as it is designed well and phased in properly, strategic regional AIs would give the world’s transportation networks a huge edge when tackling equally huge problems like climate change and suburban sprawl.
There are a few steps agencies should start taking to prepare themselves for the eventuality of strategic AI. The first is the implementation of data-driven processes on fronts that often receive less attention than operations, from project prioritisation to construction and maintenance. America’s public transportation sector has recently been experimenting with a push for data-driven funding and prioritisation decisions. The analysis required for funding applications has been increased, and projects ‘compete’ through a scoring system based on a number of sustainability metrics which push the overall system further towards sustainable design. The construction side is making a similar shift, with tablet-based inspections, 3D/4D modelling and drones moving to the forefront. All of these serve the goal of creating a system built on data and keeping that data readily available for future use.
Agencies should also focus on connected infrastructure and real-time GIS modelling to expand their passive collection capabilities. Passively gathering data is a vital step in the creation of smart regions and has been the clear direction of transportation networks for some time. Smart city initiatives are common now, but only regional agencies like state departments of transportation have the resources and jurisdiction to start building smart regions. In addition to being part of a transition to AI, these smart regions have added benefits to technologies like connected and autonomous vehicles (C/AVs) and initiatives like Mobility as a Service (MaaS). Taking a wider approach to planning is the best way to start a transition to AI-driven strategy, which means extrapolating ideas that were formerly confined to cities or even city blocks.
End goal
From an even broader perspective, public agencies around the world need to start taking a more holistic approach to mobility. The separation of road and bridge, transit, toll, and other transportation entities, is no longer an effective situation. These organisations frequently compete with each other, bickering over right of way and funding, standards and policy, and causing waste on every level of decision-making. Even if strategic AI was not inevitable, breaking down the walls between transportation agencies would still be a necessity. The public sector needs to share data, incorporate first/last mile and micromobility into the networks, and start truly looking at better mobility as a collective goal.
The end goal of all this transition and change is to implement regional strategic AIs into the transportation sector to augment the planning process. These could begin by simply making suggestions, providing data and analysis on demand, and processing a real-time model of the network using all the data the agency has available from both passive and active collection. And who knows what the future of these AIs will bring? Like the AlphaGo programs, they may come up with strategies that human designers would never have considered. They could operate on a higher level of understanding, like a child playing chess with an adult, and we may just have to trust in decisions we don’t understand. But with enormous, global problems like climate change around the corner, strategic AI may be the only enormous, global solution we have.
AlphaGo & AlphaGo Zero
Ancient Chinese board game Go is known as the most challenging classical game for artificial intelligence because of its complexity. It involves multiple layers of strategic thinking as players place their own black or white stones on a board to surround and capture their opponents’. While this seems relatively simple, there are 10 to the power of 170 possible board configurations - more than the number of atoms in the known universe – which makes Go a googol times more complex than chess.
AlphaGo is the first computer program to defeat a professional human Go player, the first to defeat a Go world champion, and is arguably the strongest Go player in history. It combines advanced search tree with deep neural networks, which take a description of the Go board as an input and process it through a number of different network layers containing millions of neuron-like connections.
AlphaGo learnt the game by playing thousands of matches with amateur and professional players. But a more advanced version, AlphaGo Zero, learnt by playing against itself, starting from completely random play – and accumulating thousands of years of human knowledge in a few days. It replaced hand-crafted rules with a deep neural network and algorithms that knew nothing beyond the basic rules. Its creative response and ability to master complex games demonstrates that a single algorithm can learn how to discover new knowledge in a range of settings.
Source: DeepMind.com