
Digital twin (DT) technology as a strategy has been widely adopted and applied to help optimise complex systems and operations in many industries, including enterprise networking, retail, healthcare, structural engineering, manufacturing, and production operations.
For transportation, Digital Twins in the Intelligent Transport Systems by Andrey Rudskoy et al, describes DT as a detailed digital reproduction of the roadway, enabling modelling and to test solutions and simulate different scenarios and events (1).
DT adoption as a strategic capability for surface transportation management has lagged behind other industries due to the limitations of data. This is especially true in applications for traffic signal control optimisation. Fortunately, the data structures and layers for signal performance measures (SPM) and adaptive signal control technologies (ASCT) applications have helped set the stage for the adoption of DT. By combining higher resolution and real-time traffic data available from new sensors, cloud-based analytics, with leading-edge simulation and modelling software technologies, DT technology - as a data-driven strategy - can be applicable for optimising traffic signal programming.
“For the first time, we have the necessary coalescence of traffic signal performance measures, machine learning and information communication technologies”
The intelligent transportation system (ITS) era ushered in the necessary information technology-based traffic management platform to establish data-driven traffic management. This platform leverages data to help transportation agencies and engineers make more informed traffic operation decisions. As a result of more and more traffic data becoming available and driving new transportation management applications, there have been many approaches to enhancing traffic signal operations.
While applications such as adaptive signal control have proven to incrementally reduce travel times and congestion along roadway segments or corridors, the traffic data resolution input and analytical capabilities have been a limiting factor in making larger efficiency gains. Until now, the industry has not experienced the coalescence of sensor, data taxonomy and analytics, distributed computing, and software technologies necessary to power real-time applications that actively optimise traffic signal control programming necessary to dynamically enhance mobility.

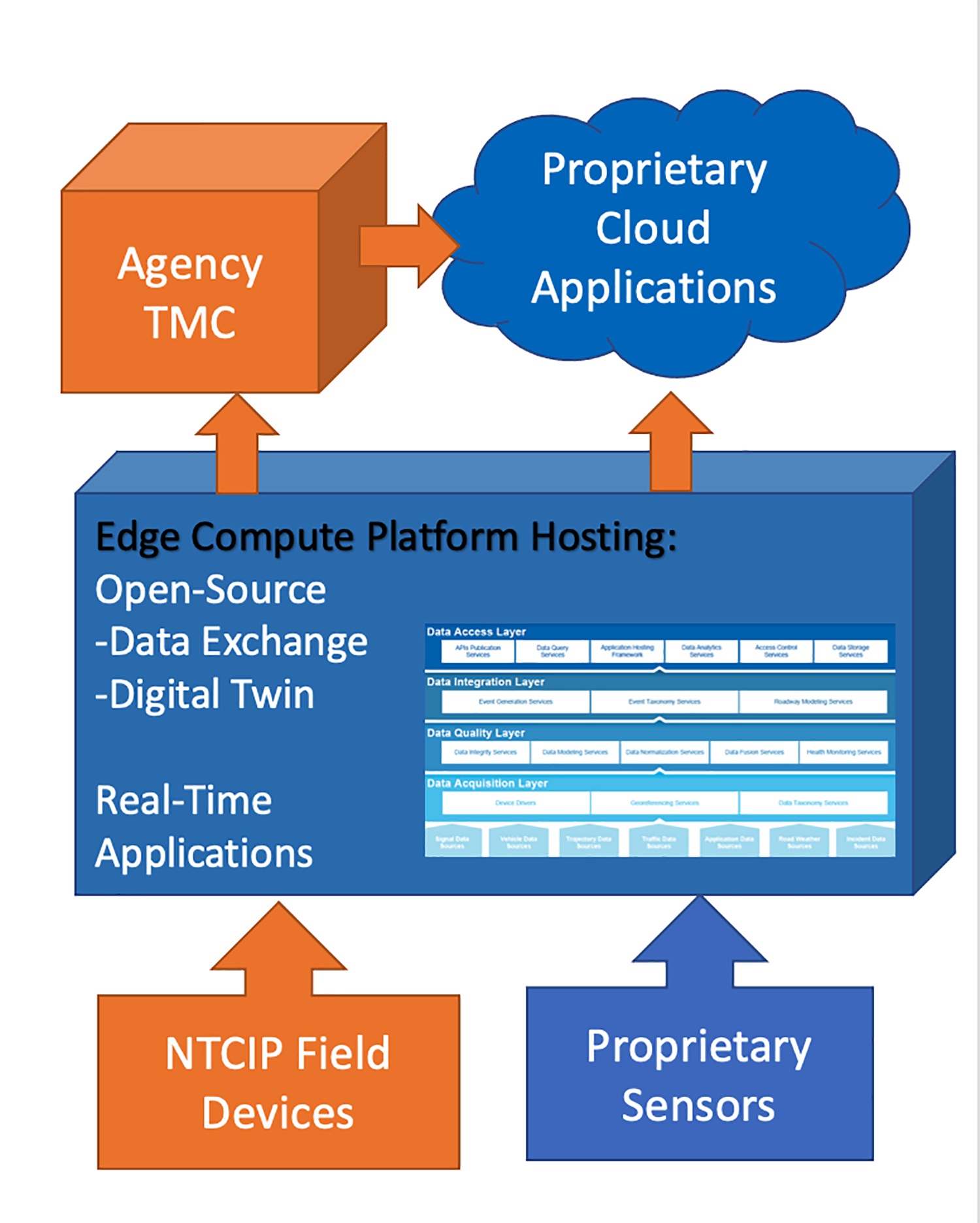
Today, the availability of new sensor and distributed or edge computing technologies provides the prospect for establishing a real-time cyber representation of vehicle traffic in a transportation network. This transportation DT represents a two-way, real-time traffic data exchange between the actual roadway and its cyber counterpart that provides the means to model, analyse and test actual traffic conditions.
Moreover, by applying the traffic data-driven platforms of signal performance measures, simulation, and modeling, as well as machine learning/artificial intelligence algorithms, there is the real potential to leverage DT as a strategy to proactively optimise traffic signal operations.
A foundation in data
ASCT and SPM, combined with a pattern optimisation capacity, have proven to incrementally reduce average wait times and improve corridor travel time reliability. For example, when traffic congestion reaches a pre-determined threshold, the adaptive signal control program is triggered to adjust the traffic signal timing manually or automatically to a pattern that better distributes green time, reduces arrivals on red, and traffic queuing at select intersections according to the current traffic conditions.
However, adaptive signal control is reactive by design and its application. As a result, it is a challenge to effectively reduce traffic delays throughout an entire transportation network for already-congested traffic conditions, including due to planned and unplanned events.
In addition, adaptive signal control has traditionally been applied only to priority corridors. While this helps to reduce travel and average wait times for vehicles where adaptive signal control is applied, cumulative average wait times can increase citywide.
To address the limitations of ASCT, as well as many other future traffic management capability issues, including connected vehicle (CV) operations, leveraging a DT strategy that runs a simulation with real-time data integration during system run-time has the potential to move traffic management optimisation from a reactive operation to proactive.
Digital twin as a strategy for traffic signal operations
Digital twin technology has the potential to fundamentally transform traffic signal management and operations. The concept of creating a DT is to help improve traffic signal operations decision making by leveraging higher resolution traffic data within an architecture that provides real-time simulation, modelling, testing, and verification.
The transportation DT can be conceptualised as traffic data being collected from different sensing and messaging systems, including deployed detection sensors, CV communication systems, personal mobile devices and CCTV cameras in real time to create an operational cyber copy of the roadway and systems.
“Digital twin technology has the potential to fundamentally transform traffic signal management and operations”
Although the concept of DT is to replicate the physical system, it is still a static representation of the roadway. For a transportation DT to be a functional and strategic tool, it must also include a mathematical modelling architecture layer on top to leverage the deployed sensor systems of the physical roadway as well as other sources of data, to be able to estimate and predict traffic and mobility interactions and user travel behaviour, ultimately to provide a representation of real-time movements of all roadway users.
A roadway DT presents a new technology paradigm in roadway traffic management strategy. In the past, traffic operation simulations and analytics - if they were utilised - occurred separately offline and used historical traffic data.
Digital twin technology raises the decision-making capability bar to the next level by enabling simulation-based control optimisation in near real-time that was technologically unattainable until now. It helps to establish a technology platform that fosters a systems operational state that can deliver real-time predictive analytics in support for new safety and optimisation strategies in traffic management. Macro and microscopic simulation results based on real-time traffic data can provide a foundation for the future real-time analysis and predictive optimisation strategies for traffic signal operations of comprehensive roadway networks.
Adopting a digital twin strategy
Real-time traffic signal optimisation and the efficacy potential of a DT platform have been made possible by new sensor and software technologies, and the high-resolution traffic data they are able to provide. One of the critical capabilities of new sensor and software technologies is the availability of vehicle classification, trajectory, tracking data, as well as data on pedestrians and bicyclists.
Driven recently by the safety applications of CV technologies, Vehicle to Vehicle (V2V) and Vehicle to Infrastructure (V2I) communications or Vehicle to Everything (V2X), the higher resolution traffic data represents continuous communication between vehicles and other roadway users with the infrastructure. This provides the fundamental physical roadway data to accurately establish a current and future state of the intersection and roadway, or situational awareness (2), which is essential to the next process step in adopting an effective DT strategy.

The importance of traffic controllers and software
In a DT-driven strategy to constantly monitor and optimise traffic flow and operations, it is necessary to create a DT of the traffic signal controllers, which requires a traffic controller firmware that provides virtual interface and control with the DT.
The DT of the traffic controllers establishes a current state of vehicles and the roadway network and provides access to the sensor and CV communications data, including object trajectories, classifications and traffic signal data.
Signal phase and timing (SPaT) and MAP (intersection geometry) messages must be transmitted from the traffic controller at the intersections to the corresponding DT. This also establishes and requires the controllers as an integral part of a distributed or edge computing network. After the traffic data is communicated from the physical controller to the DT, the process is nearly identical to existing SPM and adaptive signal control applications whereby the data is fed into the adaptive signal control algorithm that generates SPaT parameters, which are then pushed back to the physical traffic signal controller to change signal timing in response to current traffic conditions.
Unlike traditional adaptive signal control applications, the digital twin provides the capability and platform to simulate the effects of the signal change across an entire roadway network - more like a neural network instead of at a few intersections along a single priority corridor.
As a result the DT, leveraging trajectory and tracking detection data, can establish the cumulative average wait time of vehicles approaching an intersection and then the average wait time of the vehicles at intersections citywide. This can only be accomplished through the DT of the larger roadway system, including the network of traffic controllers – not just the immediate intersection(s) along a single or priority corridor.
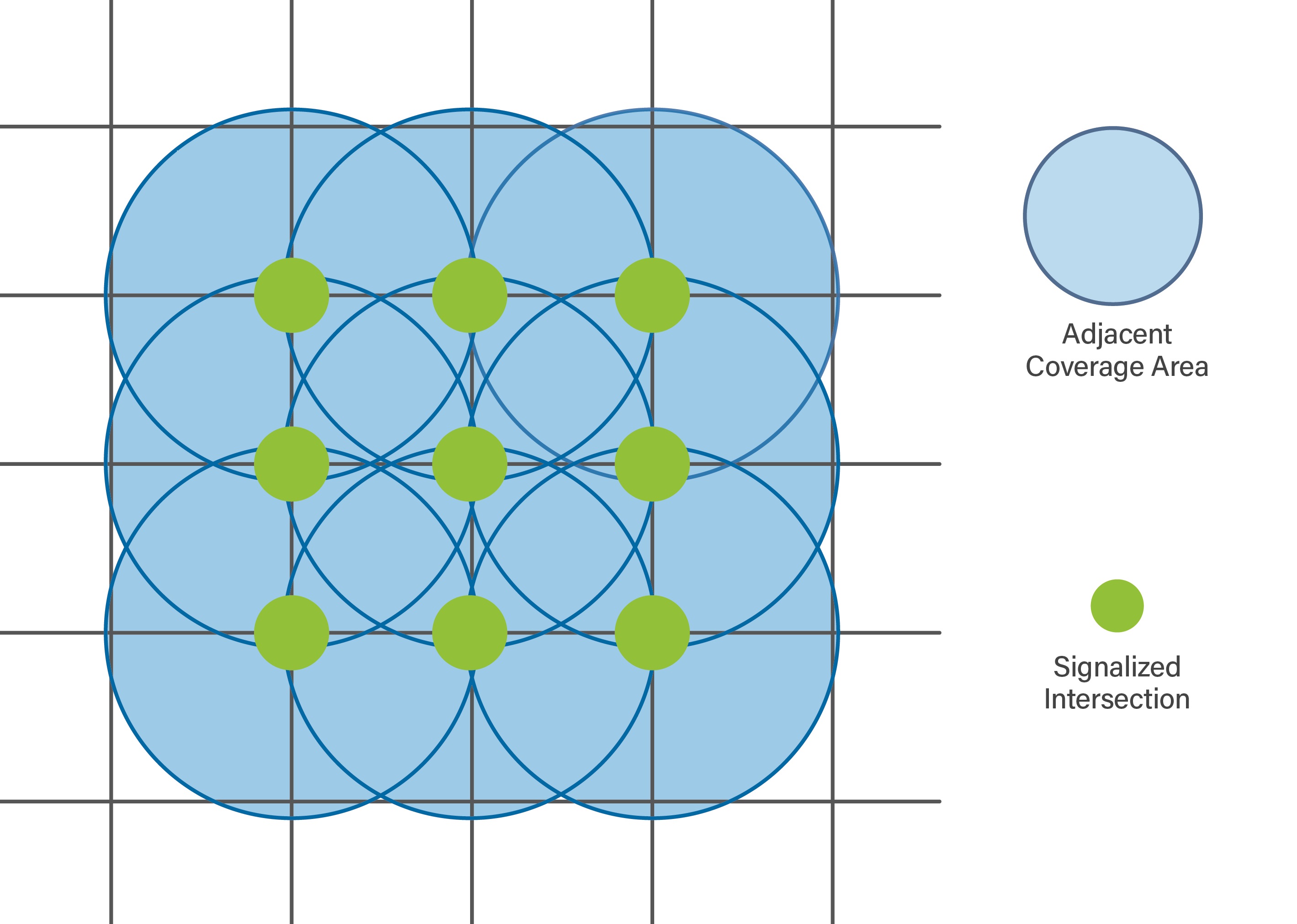
Green time can then be prioritised at intersections based on the longest average wait time for a given movement, including adjacent intersections, simulated, tested and then immediately applied. Allocating green time according to distributed average wait times throughout the roadway network can significantly reduce cumulative average wait times.
Mathematical model layer is key
The objectives of DT-driven traffic signal control operations should be able to improve mobility by:
• Reducing wait times by dynamically distributing green times
• Responding to real-time traffic demands and allocating SPaT according to cumulative average wait times
• Scaling to a citywide roadway network
• Applying predictive traffic signal control strategies
The primary challenge to leveraging a DT for traffic signal operations will be applying a mathematical model layer on top of the DT. This modelling architecture will provide the basis for macro and microscopic simulations. However, this requires combining and linking traffic data from diverse and disparate sources of the physical roadway to create a highly accurate cyber copy of the physical roadway traffic operations.
The mathematical model is key to collect and enhance information from real-time data sources to help optimise the traffic signal operations. It will aggregate real-time data feeds and real-time events, which provide information on the traffic state on the road, fusing various sources of information into one comprehensive view. This then allows the model to use a variety of analytical, simulation, and AI tools to deliver measurable traffic and mobility predictions and forecasts.
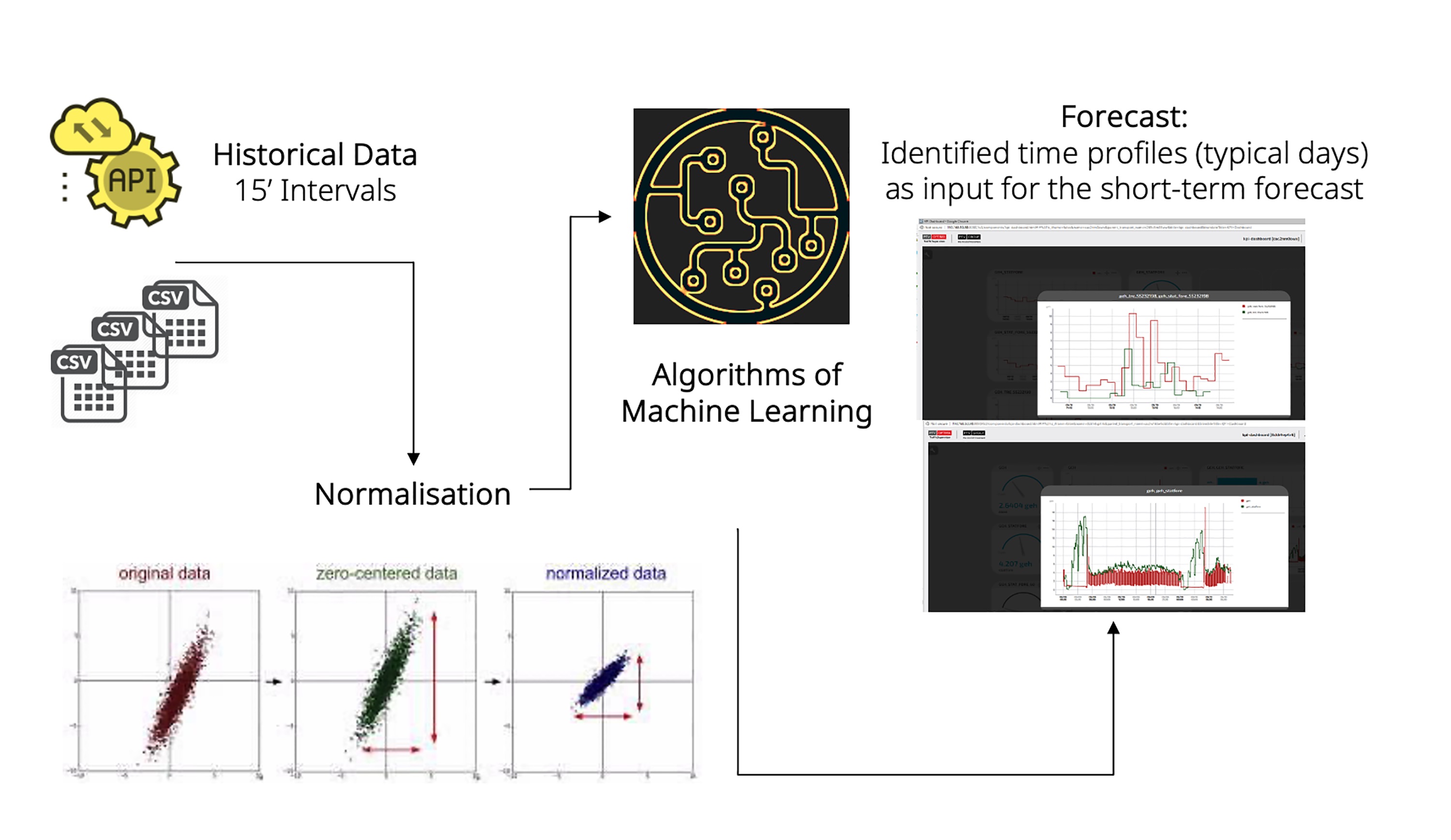
In general, predictive analytics applies various techniques including statistical inference, machine learning, data mining and data taxonomy toward the goal of dynamically and proactively optimising the signal operations through forecasting, modelling and understanding of future traffic behaviours. This methodology complements well with the architecture put in place through ITS, SPM and ASCT systems based on the aggregation and analytics of historical and real-time traffic data.
As with most highly variable operations that require process optimisation, it is also advisable to adopt an operational strategy that optimises the adaptive signal control for a range of time periods. Often referred to as a rolling-horizon (3), this strategy leverages micro and macroscopic simulation that aims to help effectively optimise a large-scale operation periodically by utilising additional data from proximate following periods. Applying this strategy for adaptive signal control will be important for predictive analytics (4).
Artificial intelligence-driven predictive traffic control algorithms that leverage the same highly accurate vehicle trajectory and tracking data developed using a rolling-horizon strategy can optimise SPaT for future traffic conditions. This can be achieved by using CV and other anonymous mobile device data.
This additional layer of high-resolution data enables the use of a phase allocation algorithm that can generate geopositional status of all roadway users to predict and proactively optimise the SPaT sequence and duration. As a result, the DT-driven adaptive signal control with predictive analytics can solve a two-level optimisation problem by prioritising and allocating traffic signal timing for a particular intersection, as well as distribute SPaT to predictively optimise travel times and efficiencies throughout an entire signalised network.
Conclusion
The introduction of DT as a strategy for traffic signal operations is expected to fundamentally change surface transportation operations. DT paired with mathematical modelling technology offers simulation-based optimisation at real time and forms the foundation for real-time predictive analytics as support for optimal traffic signal programming and safety-critical decisions in traffic management.
For the first time, we have the necessary coalescence of traffic signal performance measures, machine learning and information communication technologies to effectively adopt DT strategies that will deliver profound benefits to mobility.
ABOUT THE AUTHORS
Sunny Chakravarty is Econolite VP of engineering

Lorenzo Meschini is PTV Group VP product management & engineering real-time
References
1. A. Rudskoy, I. Ilin, and A. Prokhorov. Digital Twins in the Intelligent Transport Systems, Transportation Research Procedia, Vol. 54, 2021. ISSN:2352-1465
2. U.S. Department of Transportation (USDOT) – Raising Awareness of Artificial Intelligence for Transportation Systems Management and Operations – FHWA-HOP-19-052, December 2019.
3. L. Glomb, F. Liers, F. RÖsel. A Rolling-Horizon Approach for Multi-Period Optimization, European Journal of Operational Research, Vol. 300, Issue 1, July 2022.
4. G. Shmueli, O. Koppius. Predictive Analytics in Information Systems Research, MIS Quarterly, Vol. 35, No. 3, September 2011, Management Information Systems Research Center, University of Minnesota