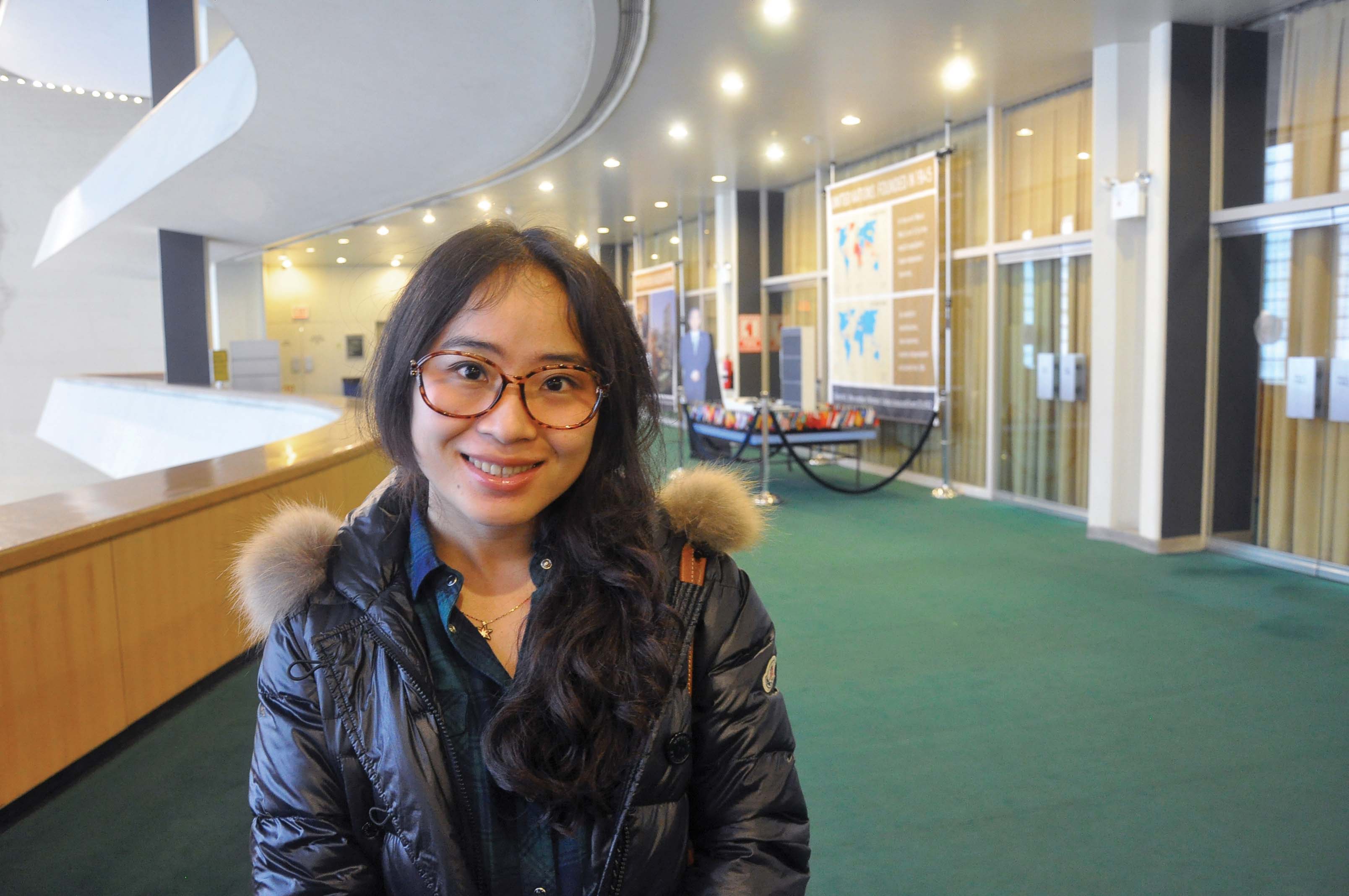
Fangce Guo
Researchers at Imperial College in London have developed a generic three-stage short-term travel prediction model that promises to give greater accuracy under both normal and abnormal conditions.
As travellers do not like the randomness of non-recurrent traffic congestion and delays, it is particularly useful for network managers to know how the ongoing traffic situation will develop when an atypical event occurs. In that way they can take appropriate actions to mitigate the delays and congestion or at least inform motorists how much of a delay they can expect. Traffic predictions in normal conditions are reasonably well catered for but predictions for abnormal conditions, whether caused by planned events or unexpected incidents, remains scientifically challenging. Therefore the main objective of researchers Fangce Guo, John Polak and Rajesh Krishnan at500 Imperial College in London was to identify the best traffic prediction framework for both normal and abnormal conditions.
Previous studies of short-term traffic prediction models tended to focus on statistical and machine learning methods and the apparent superiority of one prediction method over others when applied to a specific short-term prediction problem. Little attention has previously been paid to the issues surrounding the overall prediction model structure, in particular data smoothing and error feedback. So in developing a short-term traffic prediction model, Imperial’s researchers used a three-stage framework, including data smoothing step and error feedback mechanism, as research assistant Fangce Guo explained: “We illustrated the effect of the data smoothing and error feedback structure using five different machine learning methods: k-Nearest Neighbour approach, Grey system Model, Neural Networks (NN), Random Forests (RF) and Support Vector Regression (SVR). Not only are these methods less complicated and burdensome to implement, they may also potentially enable the prediction process to adapt more easily to normal or abnormal traffic regimes.”
The existing researches show that a wide range of methods have been used in short-term traffic prediction and highlight the apparent superiority of one prediction method over the other when applied to a particular set of circumstances. However, the accuracy of the traffic prediction using a given model depends not only on the choice of the underlying statistical or machine learning prediction tool, but also on the model structure and error feedback structure in which the prediction techniques are embedded. It has been demonstrated that simple traffic data cleaning processes can significantly improve the accuracy of traffic estimation models. This concept is also used in other disciplines such as hydrology, but to date the value of formal data smoothing techniques has not been systematically explored in the context of traffic prediction.
One of the research objectives is to develop a model that predicts traffic variables under abnormal traffic conditions, and this objective raises particular challenges. Compared with the averaged historical data, traffic patterns can suddenly change during abnormal periods resulting in parameters outside the computational window of the system.
To counter this, an error feedback mechanism that makes use of this error relationship was used to make the proposed framework dynamically self-adaptive in response to such changes, in order to maintain the accuracy of short-term traffic predictions. Therefore a data smoothing step and a mechanism of error feedback are used to create the three-stage framework for short-term traffic prediction under normal and abnormal traffic conditions.
In order to evaluate the impact of a data smoothing step and error feedback mechanism, this research used the following three different prediction frameworks for comparison in short-term traffic predictions:
Data for the study came from automatic number plate recognition (ANPR) cameras in London. Travel time data from link 1309 of the A40 road in central London (see Figure 1) were extracted in this experiment. Data from traffic under normal conditions was used in the training dataset and the testing dataset consists of known traffic incidents. Public holidays were not included in the training data but incident days were. One eastbound lane was blocked from around 17:57 to 18:40 on the testing day because of a broken down vehicle.
Travel time prediction accuracy using three traffic prediction frameworks with five machine learning methods are compared using mean percentage error (MP), mean absolute percentage error (MAPE) and root mean square error (RMSE) metrics in Figure 1.
The five methods used in this research have a similar level of prediction accuracy during normal conditions and it is clear that both data smoothing structures and feedback mechanisms can, in general, improve prediction accuracy under normal conditions.
Guo said: “Under abnormal traffic conditions, we found that the use of data smoothing and feedback structures caused a decrease in the values of MAPE metric with all five machine learning methods. This means the three-stage framework is applicable to all five machine learning methods and gave an average improvement in the MAPE value of 21.5% for short-term predictions on the A40 during the abnormal period.
“The Singular Spectrum Analysis (SSA) data smoothing structure and feedback mechanism can improve the prediction accuracy during the abnormal event period.
Moreover, the kNN based method can detect the drop in the traffic profile better than other methods and provides the best predictions.”
This improvement can be seen in Figure 2 which shows the prediction results using data from during the incident period.
Figure 3 is a scatter-plot of predicted and observed travel time data, the error auto-correlation plot of predictions, the histogram of error distribution and the time-series plot between predicted and observed travel time of the kNN method with the three-stage prediction framework. Both the data smoothing structure and feedback mechanism can improve the prediction accuracy and reduce the prediction error in short-term traffic prediction under abnormal traffic conditions using the kNN method.
In the three-stage prediction framework model the RF based method produced the most accurate predictions.
All five machine learning methods used have a similar level of prediction accuracy during normal conditions and can be improved by the two-stage traffic prediction framework. However the error feedback mechanism used in the three-stage model does not help the prediction model to significantly improve one-step ahead traffic prediction. For example, the value of MAPE by kNN using the two-stage framework for one-step ahead prediction is 3.76% which reduces to 3.66% using feedback mechanism, resulting in an improvement of 2.7%.
“These prediction results show that the three-stage framework can improve traffic prediction accuracy regardless of the machine learning method used. This is true during both normal and abnormal traffic conditions. The kNN based method showed the best ability to respond to the sudden change of traffic patterns caused by abnormal traffic events. This is because lazy learning approaches such as kNN do not involve any model construction before it is required for testing; it can quickly detect pattern changes and has the flexibility to match the best patterns from historical datasets. The findings suggest that attention should be paid to combine data smoothing and error feedback elements to arrive at a robust and accurate prediction model.
As travellers do not like the randomness of non-recurrent traffic congestion and delays, it is particularly useful for network managers to know how the ongoing traffic situation will develop when an atypical event occurs. In that way they can take appropriate actions to mitigate the delays and congestion or at least inform motorists how much of a delay they can expect. Traffic predictions in normal conditions are reasonably well catered for but predictions for abnormal conditions, whether caused by planned events or unexpected incidents, remains scientifically challenging. Therefore the main objective of researchers Fangce Guo, John Polak and Rajesh Krishnan at
Previous studies of short-term traffic prediction models tended to focus on statistical and machine learning methods and the apparent superiority of one prediction method over others when applied to a specific short-term prediction problem. Little attention has previously been paid to the issues surrounding the overall prediction model structure, in particular data smoothing and error feedback. So in developing a short-term traffic prediction model, Imperial’s researchers used a three-stage framework, including data smoothing step and error feedback mechanism, as research assistant Fangce Guo explained: “We illustrated the effect of the data smoothing and error feedback structure using five different machine learning methods: k-Nearest Neighbour approach, Grey system Model, Neural Networks (NN), Random Forests (RF) and Support Vector Regression (SVR). Not only are these methods less complicated and burdensome to implement, they may also potentially enable the prediction process to adapt more easily to normal or abnormal traffic regimes.”
The existing researches show that a wide range of methods have been used in short-term traffic prediction and highlight the apparent superiority of one prediction method over the other when applied to a particular set of circumstances. However, the accuracy of the traffic prediction using a given model depends not only on the choice of the underlying statistical or machine learning prediction tool, but also on the model structure and error feedback structure in which the prediction techniques are embedded. It has been demonstrated that simple traffic data cleaning processes can significantly improve the accuracy of traffic estimation models. This concept is also used in other disciplines such as hydrology, but to date the value of formal data smoothing techniques has not been systematically explored in the context of traffic prediction.
One of the research objectives is to develop a model that predicts traffic variables under abnormal traffic conditions, and this objective raises particular challenges. Compared with the averaged historical data, traffic patterns can suddenly change during abnormal periods resulting in parameters outside the computational window of the system.
To counter this, an error feedback mechanism that makes use of this error relationship was used to make the proposed framework dynamically self-adaptive in response to such changes, in order to maintain the accuracy of short-term traffic predictions. Therefore a data smoothing step and a mechanism of error feedback are used to create the three-stage framework for short-term traffic prediction under normal and abnormal traffic conditions.
In order to evaluate the impact of a data smoothing step and error feedback mechanism, this research used the following three different prediction frameworks for comparison in short-term traffic predictions:
- A one-stage framework which uses only one machine learning method.
- A two-stage framework that applies data smoothing step before machine learning.
- A three-stage framework which adds an error feedback mechanism to the two-stage framework.
Data for the study came from automatic number plate recognition (ANPR) cameras in London. Travel time data from link 1309 of the A40 road in central London (see Figure 1) were extracted in this experiment. Data from traffic under normal conditions was used in the training dataset and the testing dataset consists of known traffic incidents. Public holidays were not included in the training data but incident days were. One eastbound lane was blocked from around 17:57 to 18:40 on the testing day because of a broken down vehicle.
Travel time prediction accuracy using three traffic prediction frameworks with five machine learning methods are compared using mean percentage error (MP), mean absolute percentage error (MAPE) and root mean square error (RMSE) metrics in Figure 1.
The five methods used in this research have a similar level of prediction accuracy during normal conditions and it is clear that both data smoothing structures and feedback mechanisms can, in general, improve prediction accuracy under normal conditions.
Guo said: “Under abnormal traffic conditions, we found that the use of data smoothing and feedback structures caused a decrease in the values of MAPE metric with all five machine learning methods. This means the three-stage framework is applicable to all five machine learning methods and gave an average improvement in the MAPE value of 21.5% for short-term predictions on the A40 during the abnormal period.
“The Singular Spectrum Analysis (SSA) data smoothing structure and feedback mechanism can improve the prediction accuracy during the abnormal event period.
Moreover, the kNN based method can detect the drop in the traffic profile better than other methods and provides the best predictions.”
This improvement can be seen in Figure 2 which shows the prediction results using data from during the incident period.
Figure 3 is a scatter-plot of predicted and observed travel time data, the error auto-correlation plot of predictions, the histogram of error distribution and the time-series plot between predicted and observed travel time of the kNN method with the three-stage prediction framework. Both the data smoothing structure and feedback mechanism can improve the prediction accuracy and reduce the prediction error in short-term traffic prediction under abnormal traffic conditions using the kNN method.
In the three-stage prediction framework model the RF based method produced the most accurate predictions.
All five machine learning methods used have a similar level of prediction accuracy during normal conditions and can be improved by the two-stage traffic prediction framework. However the error feedback mechanism used in the three-stage model does not help the prediction model to significantly improve one-step ahead traffic prediction. For example, the value of MAPE by kNN using the two-stage framework for one-step ahead prediction is 3.76% which reduces to 3.66% using feedback mechanism, resulting in an improvement of 2.7%.
“These prediction results show that the three-stage framework can improve traffic prediction accuracy regardless of the machine learning method used. This is true during both normal and abnormal traffic conditions. The kNN based method showed the best ability to respond to the sudden change of traffic patterns caused by abnormal traffic events. This is because lazy learning approaches such as kNN do not involve any model construction before it is required for testing; it can quickly detect pattern changes and has the flexibility to match the best patterns from historical datasets. The findings suggest that attention should be paid to combine data smoothing and error feedback elements to arrive at a robust and accurate prediction model.