Innovative use of fleet management-generated data has optimised passenger service running times and achieved full payback in its first quarter Metro Vancouver’s South Coast British Columbia Transportation Authority (TransLink) has gained substantial benefits in bus idle time savings from a business intelligence (BI) solution, built from data captured in its ITS-based fleet management system. Delivered by public transport ITS specialist Init under a contract awarded in 2006, this includes on-board computers,
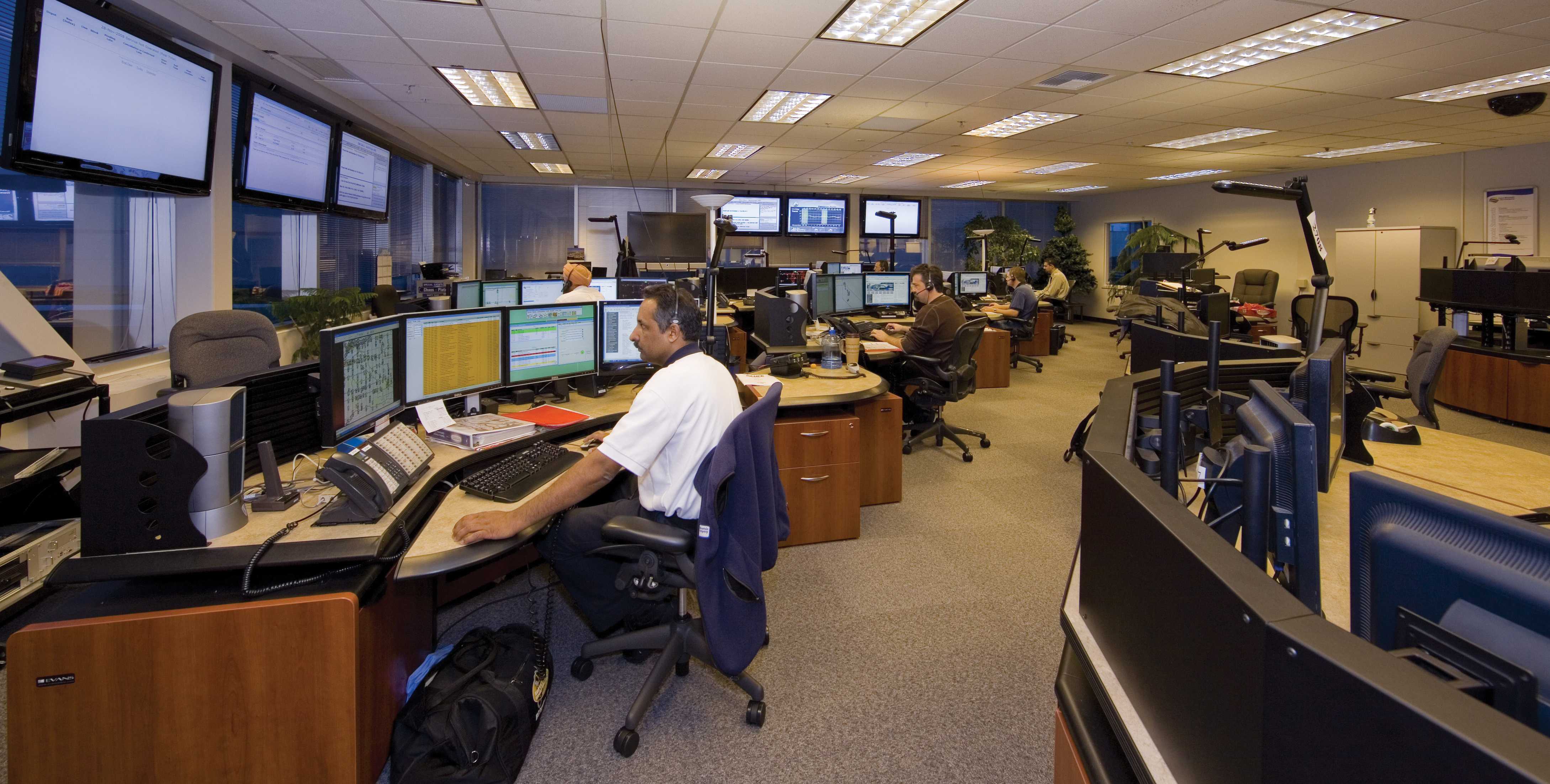
The TransLink Control Centre
Innovative use of fleet management-generated data has optimised passenger service running times and achieved full payback in its first quarter
Metro Vancouver’s South Coast British Columbia Transportation Authority (For a network of this scale, with measuring service efficiency as a primary concern, gaining effective access to delivered service data was proving critical for analysing the quality of operations, and, in turn, creating more efficient bus schedules.
Init’s system was capturing the necessary data. However, the agency needed to access it more quickly and conveniently for comparison with current schedule data on trips, and timing point segments and stops. This would enable it effectively to analyse each run time (the time taken to complete a journey along a scheduled route) and make service adjustments in response.
Prior to 2012, service analysts with the agency’s Coast Mountain Bus Company (CMBC) subsidiary, which operates 200 of its 220 bus routes, were – when evaluating revenue service run times quarterly prior to introducing a new schedule - using a process that took between one to 12 hours per route. Apart from the staff hours consumed, this limited the opportunities, for all but priority routes, to make service adjustments to reduce operating costs.
High performance data
The solution envisaged was to create a high-performance data warehouse where delivered service data could be married to static schedule data for analysing variations and improving run time efficiency. In October 2010, TransLink project manager Darla Jamieson prepared a business case for a run time analysis project that would extract and process the critical data more efficiently and give useful insights into where services could be streamlined.Says project sponsor and CMBC director of transit service design Tom Fink: “We needed to optimise our existing driver and bus resources by making the best use of our available data.” The project’s four main targets were to:
- Enable faster access to, and analysis of, delivered service data;
- Remove idle times from bus schedules;
- Enable faster reallocation of, or adjustment to, services where needed; and
- Tell a success story about the importance of the data.
year reThe TransLink team approached the challenge as a BI project, rather than a traditional systems development one. (One key difference is that, in the former, the focus is on how the business uses, perceives, and questions the data. Clear definitions and consistent terminology are crucial).
The team studied current and future analytical requirements and integration points between service-related data from all sources - although, says Jamieson, “it was equally important to keep our eye on the prize - the run time analysis process. This was the baseline we used to determine which data carried the most weight, was worth the effort to profile and cleanse, and would therefore justify expensive transformations in the data warehouse.”
On the importance of the next step, data profiling, she continues: “You need to undertake this on the raw source data to understand exactly how it is captured and how operating behaviours impact it. This process took several months and substantially more effort than originally anticipated.”
Following the design phase, TransLink approved a change request to acquire specialised data warehousing hardware from Hewlett Packard when it transpired that existing infrastructure could not deliver the performance needed for the estimated transactional volumes and data retention needs. The hardware improved the productivity of the project developers and testers with faster turnarounds of extract-transform-load (ETL) code changes.
TransLink also provided additional time to ensure that the data was being captured correctly on the road. “The way you build your schedules, and define your garage gate and stop coordinates, and the way the data warehouse operators are trained to use the system, all impact the quality of the data available for analysis,” says Jamieson.
A main goal
With the ability to spot idle times as a main goal, identification of excess run times (where the time taken to travel between two points is consistently at least two minutes less than that allocated in the schedule - tempered by the unique characteristics of the route) proved significant in helping create greater efficiency within schedules.A secondary goal was to confirm suspicions that more idle time would be found within garage deadheads (the non-revenue re-positionings of buses from garages to the starting points of revenue services). This was previously unverifiable because the relevant data had never been available for analysis prior to the warehouse.
With so much attention focused on getting to the data, the team realised how much a proactive approach during the original fleet management system implementation could have helped.
Gerry Akkerman, TransLink director of business technology planning, served on the project steering committee and found it innovative. He says: “Agencies need to think about how they want to use their data from the outset of their ITS implementation.
We learned very quickly that full access to data sets was absolutely necessary and that trying to clean data up or to understand its architecture after system implementation was a heavy lift.”
Results
The project concluded in 21 months, using 28 TransLink and Init staff, with satisfactory results. The service data from the warehouse has proved more than sufficient to deliver the value needed and achieved full payback in one quarter. Analysts can now extract and compile data at an average rate of less than five minutes per route - a huge time saving. They have increased the number of route analyses from 50 per quarter to 1,200, including all routes in both directions on all service days.With high-speed data access, schedulers are now saving over 36,000 hours of idle time annually – 720% of the agency’s target of 5,000 hours. Fink, a strong advocate of BI, concludes: “The project has not only enabled us to optimise running times, it has also improved union-management relations by allowing us to target problem areas and resolve issues quickly. Our efficiency has greatly improved and there are gains yet to come.”
“Beyond the obvious tangible returns,” says Init CEO Roland Staib, “there is intrinsic value in the mined computer-aided dispatch and automatic vehicle location (CAD/AVL) data - not just for streamlining services, but also for future BI applications.” The agency is using charts and other visualisations as an outreach tool for sharing with bus drivers how run times are analysed.
On the basis of service adjustments made in the first year, the project will deliver an internal rate of return of 178% over five years. The key to its success was output from the Init fleet management system. Says Akkerman: “We took the Init data and turned it into insight.”
Previous | Target | Achieved | |
---|---|---|---|
Analyses per quarter | 50 | 200 | 1,200 |
Data extract time per route | 1-12 hours | <1 hour | <5 minutes |
Idle time in schedules | Excessive; needing removal for reallocation to service improvements or new services | 5,000 hours per year reduction | 36,770 hours per year reduction: 23,000 hours run time (revenue); 13,750 hours deadheads (non-revenue) |