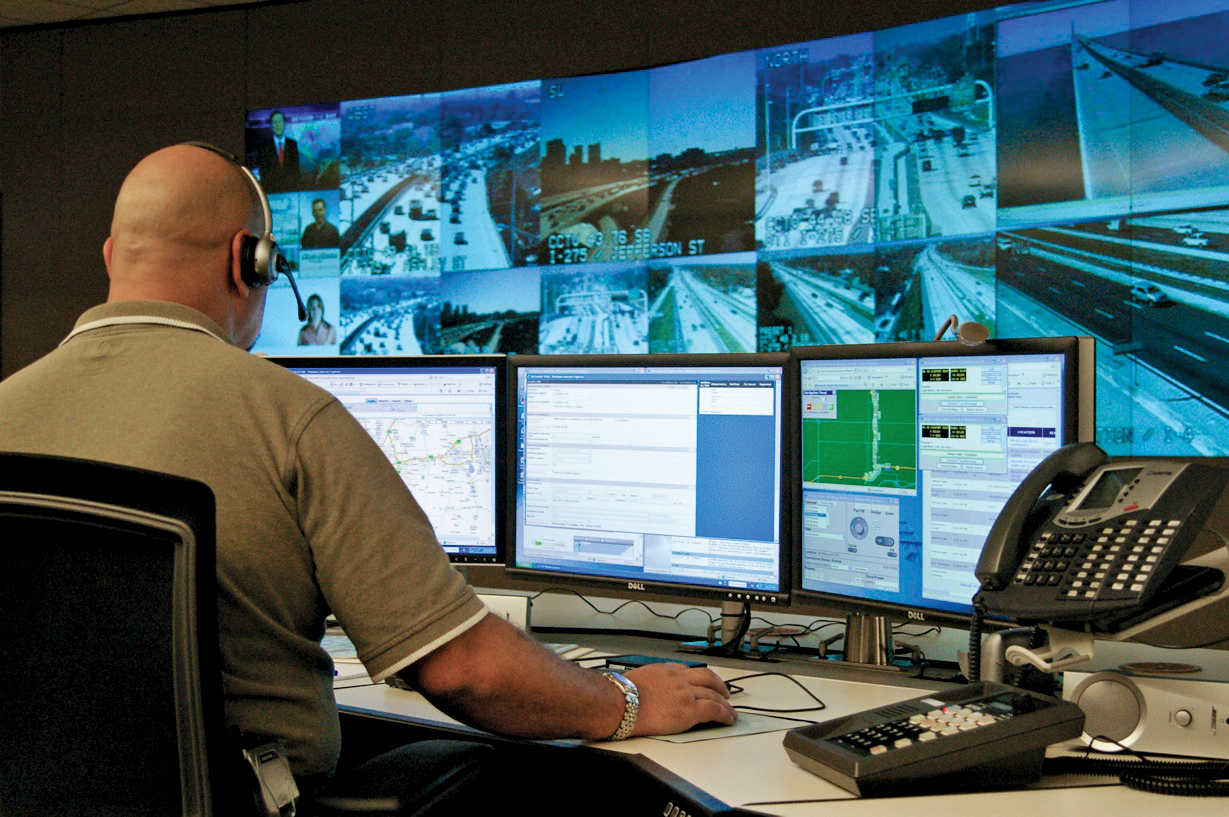
Astronomical is not too grand a term to describe the current rate of growth in transportation-related data. Massive amounts of traffic related information, such as speed, volume, incidents and weather are being generated every second by road operators and users alike.
Big data’ derives its name from the sheer amount and complexity of available raw data. Its potential value is starting to emerge among the intelligent transportation systems community. A gold rush is taking place to capture this value, with data mining at the forefront. Broadly understood, data mining is a process that identifies useful patterns in the data. Software tools resolve patterns to create models, which can then be used to replicate current conditions and predict future trends and behaviours in a process known as predictive analytics. The benefits of data mining can be readily realised in the transportation field for road operators, traffic engineers, planners, emergency managers and others alike. Data mining is also about gaining efficiencies in planning and operations. For example, a road operator would normally use traffic volume, speed and ‘eye-ball’ staff observations to identify recurring and non-reoccurring congestion for optimisation of traffic signal timing. Yet, the optimisation of traffic flow is highly dependent on empirical models due to the poor correlation of theoretical models to actual observed traffic flows.Data mining can increase the correlation between theoretical and real-life congestion by associating traffic volume and speed to sudden speed changes, accidents, work or school schedules, or weather changes. Combining traffic data with other variables outside conventional theoretical models, data mining may strike a deep, rich vein – pointing to patterns of recurring congestion on a level that may not have been discovered otherwise. From that point, predictive analytics can support improved, proactive traffic operations, such as adaptive signal control, variable speed limits, traffic advice and dynamic congestion pricing – processes designed to smooth disturbances and reduce congestion. But for data mining to be useful, highway authorities must tap into new and unconventional data sources. Vehicle probe and telemetry data, as well as other ‘user generated’ data needs to be collected and mined. An information gold mine? If data mining is the process whereby useful information is extracted from data sets, data warehouses are where the raw data is aggregated and hosted. Data warehouses are databases that contain standardised raw data amalgamated from multiple information sources. Inside these warehouses, any semantic discrepancies in data collected across source systems are resolved; historical data is preserved, annotated and archived.
Operations engineers, planners, and researchers can apply data-mining software tools to test hypotheses and find patterns in the data, providing valuable strategic insights for decision making. These warehouses are not easily established. Data from multiple sources are collected, they must be painstakingly integrated and normalised before they can be stored in the warehouse. Furthermore, it is important that data warehouses contain standard interfaces so applications from varying devices and systems can append, query and update the data securely. What is the benefit for road operators? In short, warehouses can aggregate and standardise certain types of data beyond the capabilities of typical Advanced Traffic Management Systems (ATMS). Data traditionally beyond road operators’ reach include passenger vehicle telemetry, truck/freight, transit, parking and even weather data.
Even unstructured or unconventional data sources may be queried and incorporated into a warehouse, such as social network feeds or user-generated ‘crowdsourced’ incident reports. All of this will give road operators a more holistic view of their traffic networks, to allocate resources more efficiently (eg, provide additional transit capacity when needed) or fine tune traffic controls. Or a data warehouse? Data warehouses vary tremendously in size and purpose. Larger national-level warehouses for transportation tend to cater for the research community, while data warehouses for transportation at the regional level are more suited to urban and corridor road operators.
For example, ‘Smart City’ analytics tools attempt to give metropolitan transportation operators a holistic view of urban mobility and generally require data collected from sources within or immediately surrounding the metropolitan area of interest. Data from areas miles away may be completely extraneous. However, a larger data warehouse that includes several types of data from many regions may be of great interest to researchers and application developers, because of the possible knowledge gained from recognising inter-regional or even international patterns.
There are prominent examples of regional data warehouses in the US, such as
Gold in store
Private sector firms have been active in developing products to help transportation decision makers realise the benefits of data warehousing and mining. Traffic information providerCompanies like
ParkMe warehouses historical data for thousands of facilities to show users two weeks in advance where to find available parking in a given area. ParkMe and others then collect real-time parking occupancy data to reflect changes that may buck historical trends, identifying empty parking spaces for drivers when contingencies occur, such as a major unscheduled public event.The gold rush for transportation data is particularly real for urban planning and management.
City authorities are beginning to harness valuable knowledge created as a result of data warehousing and mining applications. Intelligent infrastructure aims to centralise information flows and warehouse everything from traffic data to water flow and criminal activity logs. Analytics are needed; ones that help managers decide where to deploy on-duty police officers in order to reduce drunk driving, where to concentrate road maintenance dollars to improve safety, or how to price tolling, transit and parking in a coordinated, equitable way.
Striking it Rich
Smarter cars will generate terabytes of data while the scale of transportation data will rise to the order of petabytes. Examples of the potential of vehicle data mining abound. For instance, mining of windshield wiper and other vehicle data could be used to refine reporting and forecasting of road weather conditions. Crash avoidance features in cars are coming and telematics services such asThis article was written by Adrian Guan, Sean Murphy, Patrick Son and Steven Bayless of
Urban development and growth require smart transportation solutions and in that vein, ITS America has been holding a series of symposia focusing on intelligent infrastructure. In March this year, ITS America hosted a Smart Parking Symposium along with the California Department of Transportation (Caltrans), the San Francisco Bay Area Metropolitan Transportation Commission (MTC) and the Green Parking Council. In July, ITS America will host the “Complete Streets” Symposium with the City of Chicago.